Estimating effect of multiple treatments#
[1]:
from dowhy import CausalModel
import dowhy.datasets
import warnings
warnings.filterwarnings('ignore')
[2]:
data = dowhy.datasets.linear_dataset(10, num_common_causes=4, num_samples=10000,
num_instruments=0, num_effect_modifiers=2,
num_treatments=2,
treatment_is_binary=False,
num_discrete_common_causes=2,
num_discrete_effect_modifiers=0,
one_hot_encode=False)
df=data['df']
df.head()
[2]:
X0 | X1 | W0 | W1 | W2 | W3 | v0 | v1 | y | |
---|---|---|---|---|---|---|---|---|---|
0 | 0.439382 | -0.205453 | 2.529354 | 1.113537 | 2 | 0 | 13.771004 | 13.000752 | 472.597362 |
1 | 0.149839 | -2.079429 | -1.324029 | -0.382790 | 2 | 3 | 20.099593 | 12.052128 | -1533.635739 |
2 | -1.274413 | -0.251587 | -1.933019 | 0.624964 | 0 | 0 | -2.515991 | -0.752427 | -52.169030 |
3 | -0.143148 | 0.981800 | 0.062478 | 0.157607 | 3 | 2 | 22.405199 | 16.950783 | 1669.945671 |
4 | -0.518465 | -2.574587 | -0.364611 | -0.051063 | 3 | 1 | 13.886915 | 14.532450 | -2206.748459 |
[3]:
model = CausalModel(data=data["df"],
treatment=data["treatment_name"], outcome=data["outcome_name"],
graph=data["gml_graph"])
[4]:
model.view_model()
from IPython.display import Image, display
display(Image(filename="causal_model.png"))
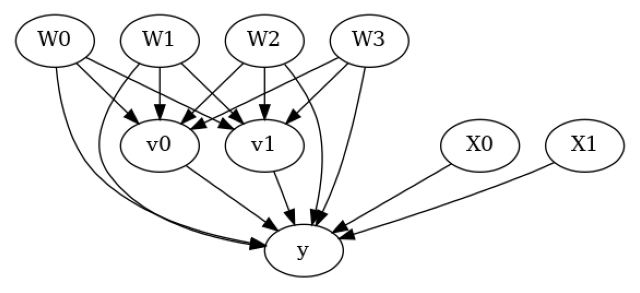
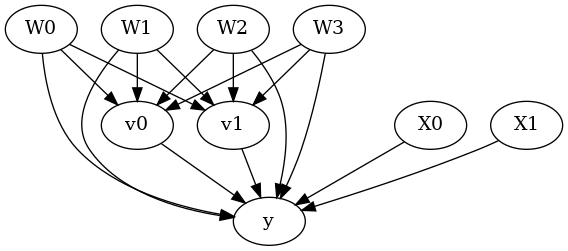
[5]:
identified_estimand= model.identify_effect(proceed_when_unidentifiable=True)
print(identified_estimand)
Estimand type: EstimandType.NONPARAMETRIC_ATE
### Estimand : 1
Estimand name: backdoor
Estimand expression:
d
─────────(E[y|W0,W1,W3,W2])
d[v₀ v₁]
Estimand assumption 1, Unconfoundedness: If U→{v0,v1} and U→y then P(y|v0,v1,W0,W1,W3,W2,U) = P(y|v0,v1,W0,W1,W3,W2)
### Estimand : 2
Estimand name: iv
No such variable(s) found!
### Estimand : 3
Estimand name: frontdoor
No such variable(s) found!
Linear model#
Let us first see an example for a linear model. The control_value and treatment_value can be provided as a tuple/list when the treatment is multi-dimensional.
The interpretation is change in y when v0 and v1 are changed from (0,0) to (1,1).
[6]:
linear_estimate = model.estimate_effect(identified_estimand,
method_name="backdoor.linear_regression",
control_value=(0,0),
treatment_value=(1,1),
method_params={'need_conditional_estimates': False})
print(linear_estimate)
*** Causal Estimate ***
## Identified estimand
Estimand type: EstimandType.NONPARAMETRIC_ATE
### Estimand : 1
Estimand name: backdoor
Estimand expression:
d
─────────(E[y|W0,W1,W3,W2])
d[v₀ v₁]
Estimand assumption 1, Unconfoundedness: If U→{v0,v1} and U→y then P(y|v0,v1,W0,W1,W3,W2,U) = P(y|v0,v1,W0,W1,W3,W2)
## Realized estimand
b: y~v0+v1+W0+W1+W3+W2+v0*X0+v0*X1+v1*X0+v1*X1
Target units: ate
## Estimate
Mean value: -100.73971170137605
You can estimate conditional effects, based on effect modifiers.
[7]:
linear_estimate = model.estimate_effect(identified_estimand,
method_name="backdoor.linear_regression",
control_value=(0,0),
treatment_value=(1,1))
print(linear_estimate)
*** Causal Estimate ***
## Identified estimand
Estimand type: EstimandType.NONPARAMETRIC_ATE
### Estimand : 1
Estimand name: backdoor
Estimand expression:
d
─────────(E[y|W0,W1,W3,W2])
d[v₀ v₁]
Estimand assumption 1, Unconfoundedness: If U→{v0,v1} and U→y then P(y|v0,v1,W0,W1,W3,W2,U) = P(y|v0,v1,W0,W1,W3,W2)
## Realized estimand
b: y~v0+v1+W0+W1+W3+W2+v0*X0+v0*X1+v1*X0+v1*X1
Target units:
## Estimate
Mean value: -100.73971170137605
### Conditional Estimates
__categorical__X0 __categorical__X1
(-4.284000000000001, -1.409] (-5.128, -1.633] -283.778661
(-1.633, -1.053] -227.693425
(-1.053, -0.525] -195.630781
(-0.525, 0.0461] -159.069413
(0.0461, 2.616] -104.503596
(-1.409, -0.819] (-5.128, -1.633] -226.424752
(-1.633, -1.053] -170.983067
(-1.053, -0.525] -135.112545
(-0.525, 0.0461] -102.557610
(0.0461, 2.616] -43.652254
(-0.819, -0.329] (-5.128, -1.633] -191.453361
(-1.633, -1.053] -135.394258
(-1.053, -0.525] -100.786258
(-0.525, 0.0461] -65.979753
(0.0461, 2.616] -9.941882
(-0.329, 0.276] (-5.128, -1.633] -156.472730
(-1.633, -1.053] -100.336103
(-1.053, -0.525] -63.620204
(-0.525, 0.0461] -31.116636
(0.0461, 2.616] 25.088725
(0.276, 3.165] (-5.128, -1.633] -99.093020
(-1.633, -1.053] -43.450080
(-1.053, -0.525] -4.915625
(-0.525, 0.0461] 26.443889
(0.0461, 2.616] 82.101963
dtype: float64
More methods#
You can also use methods from EconML or CausalML libraries that support multiple treatments. You can look at examples from the conditional effect notebook: https://py-why.github.io/dowhy/example_notebooks/dowhy-conditional-treatment-effects.html
Propensity-based methods do not support multiple treatments currently.