Mediation analysis with DoWhy: Direct and Indirect Effects#
[1]:
import numpy as np
import pandas as pd
from dowhy import CausalModel
import dowhy.datasets
# Warnings and logging
import warnings
warnings.filterwarnings('ignore')
Creating a dataset#
[2]:
# Creating a dataset with a single confounder and a single mediator (num_frontdoor_variables)
data = dowhy.datasets.linear_dataset(10, num_common_causes=1, num_samples=10000,
num_instruments=0, num_effect_modifiers=0,
num_treatments=1,
num_frontdoor_variables=1,
treatment_is_binary=False,
outcome_is_binary=False)
df = data['df']
print(df.head())
FD0 W0 v0 y
0 -5.161805 -0.238899 -2.774685 -24.838799
1 -5.297022 -0.639080 -2.613453 -27.150863
2 -21.917294 -2.305822 -10.291745 -110.928846
3 -5.663202 -0.588138 -2.992840 -28.633400
4 -4.601948 -0.892113 -2.831342 -25.002046
Step 1: Modeling the causal mechanism#
We create a dataset following a causal graph based on the frontdoor criterion. That is, there is no direct effect of the treatment on outcome; all effect is mediated through the frontdoor variable FD0.
[3]:
model = CausalModel(df,
data["treatment_name"],data["outcome_name"],
data["gml_graph"],
missing_nodes_as_confounders=True)
model.view_model()
from IPython.display import Image, display
display(Image(filename="causal_model.png"))
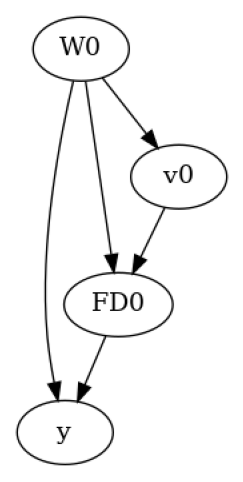
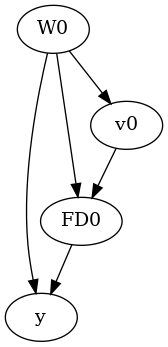
Step 2: Identifying the natural direct and indirect effects#
We use the estimand_type
argument to specify that the target estimand should be for a natural direct effect or the natural indirect effect. For definitions, see Interpretation and Identification of Causal Mediation by Judea Pearl.
Natural direct effect: Effect due to the path v0->y
Natural indirect effect: Effect due to the path v0->FD0->y (mediated by FD0).
[4]:
# Natural direct effect (nde)
identified_estimand_nde = model.identify_effect(estimand_type="nonparametric-nde",
proceed_when_unidentifiable=True)
print(identified_estimand_nde)
Estimand type: EstimandType.NONPARAMETRIC_NDE
### Estimand : 1
Estimand name: mediation
Estimand expression:
⎡ d ⎤
E⎢─────(y|FD0)⎥
⎣d[v₀] ⎦
Estimand assumption 1, Mediation: FD0 intercepts (blocks) all directed paths from v0 to y except the path {v0}→{y}.
Estimand assumption 2, First-stage-unconfoundedness: If U→{v0} and U→{FD0} then P(FD0|v0,U) = P(FD0|v0)
Estimand assumption 3, Second-stage-unconfoundedness: If U→{FD0} and U→y then P(y|FD0, v0, U) = P(y|FD0, v0)
[5]:
# Natural indirect effect (nie)
identified_estimand_nie = model.identify_effect(estimand_type="nonparametric-nie",
proceed_when_unidentifiable=True)
print(identified_estimand_nie)
Estimand type: EstimandType.NONPARAMETRIC_NIE
### Estimand : 1
Estimand name: mediation
Estimand expression:
⎡ d d ⎤
E⎢──────(y)⋅─────([FD₀])⎥
⎣d[FD₀] d[v₀] ⎦
Estimand assumption 1, Mediation: FD0 intercepts (blocks) all directed paths from v0 to y except the path {v0}→{y}.
Estimand assumption 2, First-stage-unconfoundedness: If U→{v0} and U→{FD0} then P(FD0|v0,U) = P(FD0|v0)
Estimand assumption 3, Second-stage-unconfoundedness: If U→{FD0} and U→y then P(y|FD0, v0, U) = P(y|FD0, v0)
Step 3: Estimation of the effect#
Currently only two stage linear regression is supported for estimation. We plan to add a non-parametric Monte Carlo method soon as described in Imai, Keele and Yamamoto (2010).
Natural Indirect Effect#
The estimator converts the mediation effect estimation to a series of backdoor effect estimations. 1. The first-stage model estimates the effect from treatment (v0) to the mediator (FD0). 2. The second-stage model estimates the effect from mediator (FD0) to the outcome (Y).
[6]:
import dowhy.causal_estimators.linear_regression_estimator
causal_estimate_nie = model.estimate_effect(identified_estimand_nie,
method_name="mediation.two_stage_regression",
confidence_intervals=False,
test_significance=False,
method_params = {
'first_stage_model': dowhy.causal_estimators.linear_regression_estimator.LinearRegressionEstimator,
'second_stage_model': dowhy.causal_estimators.linear_regression_estimator.LinearRegressionEstimator
}
)
print(causal_estimate_nie)
*** Causal Estimate ***
## Identified estimand
Estimand type: EstimandType.NONPARAMETRIC_NIE
### Estimand : 1
Estimand name: mediation
Estimand expression:
⎡ d d ⎤
E⎢──────(y)⋅─────([FD₀])⎥
⎣d[FD₀] d[v₀] ⎦
Estimand assumption 1, Mediation: FD0 intercepts (blocks) all directed paths from v0 to y except the path {v0}→{y}.
Estimand assumption 2, First-stage-unconfoundedness: If U→{v0} and U→{FD0} then P(FD0|v0,U) = P(FD0|v0)
Estimand assumption 3, Second-stage-unconfoundedness: If U→{FD0} and U→y then P(y|FD0, v0, U) = P(y|FD0, v0)
## Realized estimand
(b: FD0~v0+W0)*(b: y~FD0+W0)
Target units: ate
## Estimate
Mean value: 8.854778256842833
Note that the value equals the true value of the natural indirect effect (up to random noise).
[7]:
print(causal_estimate_nie.value, data["ate"])
8.854778256842833 8.961633713652406
The parameter is called ate
because in the simulated dataset, the direct effect is set to be zero.
Natural Direct Effect#
Now let us check whether the direct effect estimator returns the (correct) estimate of zero.
[8]:
causal_estimate_nde = model.estimate_effect(identified_estimand_nde,
method_name="mediation.two_stage_regression",
confidence_intervals=False,
test_significance=False,
method_params = {
'first_stage_model': dowhy.causal_estimators.linear_regression_estimator.LinearRegressionEstimator,
'second_stage_model': dowhy.causal_estimators.linear_regression_estimator.LinearRegressionEstimator
}
)
print(causal_estimate_nde)
*** Causal Estimate ***
## Identified estimand
Estimand type: EstimandType.NONPARAMETRIC_NDE
### Estimand : 1
Estimand name: mediation
Estimand expression:
⎡ d ⎤
E⎢─────(y|FD0)⎥
⎣d[v₀] ⎦
Estimand assumption 1, Mediation: FD0 intercepts (blocks) all directed paths from v0 to y except the path {v0}→{y}.
Estimand assumption 2, First-stage-unconfoundedness: If U→{v0} and U→{FD0} then P(FD0|v0,U) = P(FD0|v0)
Estimand assumption 3, Second-stage-unconfoundedness: If U→{FD0} and U→y then P(y|FD0, v0, U) = P(y|FD0, v0)
## Realized estimand
(b: y~v0+W0) - ((b: FD0~v0+W0)*(b: y~FD0+W0))
Target units: ate
## Estimate
Mean value: -3.29335893400895e-05
Step 4: Refutations#
TODO