Confounding Example: Finding causal effects from observed data#
Suppose you are given some data with treatment and outcome. Can you determine whether the treatment causes the outcome, or the correlation is purely due to another common cause?
[1]:
import numpy as np
import pandas as pd
import matplotlib.pyplot as plt
import math
import dowhy
from dowhy import CausalModel
import dowhy.datasets, dowhy.plotter
# Config dict to set the logging level
import logging.config
DEFAULT_LOGGING = {
'version': 1,
'disable_existing_loggers': False,
'loggers': {
'': {
'level': 'INFO',
},
}
}
logging.config.dictConfig(DEFAULT_LOGGING)
Let’s create a mystery dataset for which we need to determine whether there is a causal effect.#
Creating the dataset. It is generated from either one of two models: * Model 1: Treatment does cause outcome. * Model 2: Treatment does not cause outcome. All observed correlation is due to a common cause.
[2]:
rvar = 1 if np.random.uniform() >0.5 else 0
data_dict = dowhy.datasets.xy_dataset(10000, effect=rvar,
num_common_causes=1,
sd_error=0.2)
df = data_dict['df']
print(df[["Treatment", "Outcome", "w0"]].head())
Treatment Outcome w0
0 7.588773 15.439320 1.483548
1 8.464945 17.079393 2.464048
2 1.985935 4.099270 -3.865950
3 7.118398 13.774239 0.746508
4 3.870168 7.347380 -2.322948
[3]:
dowhy.plotter.plot_treatment_outcome(df[data_dict["treatment_name"]], df[data_dict["outcome_name"]],
df[data_dict["time_val"]])
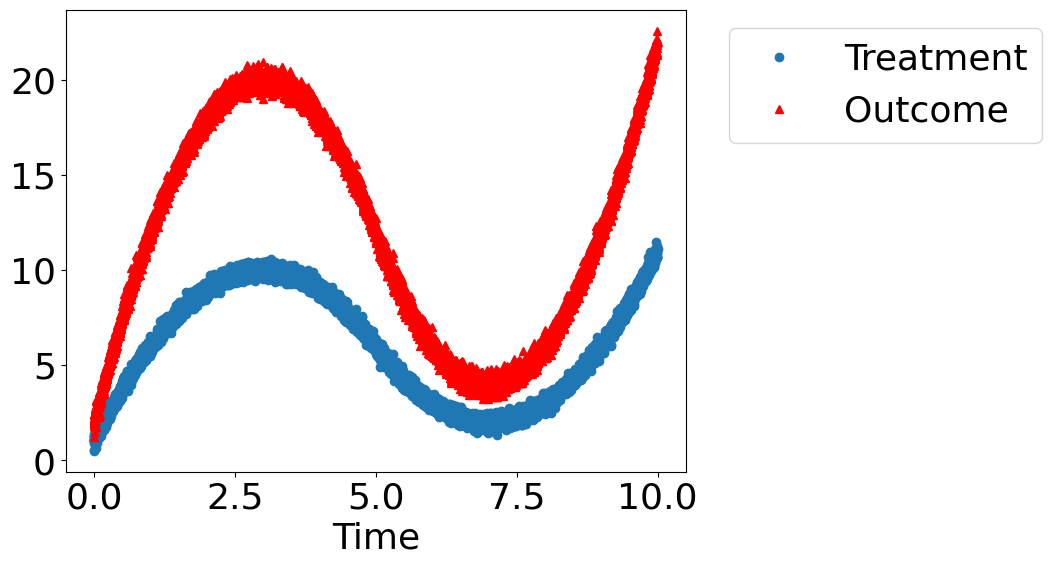
Using DoWhy to resolve the mystery: Does Treatment cause Outcome?#
STEP 1: Model the problem as a causal graph#
Initializing the causal model.
[4]:
model= CausalModel(
data=df,
treatment=data_dict["treatment_name"],
outcome=data_dict["outcome_name"],
common_causes=data_dict["common_causes_names"],
instruments=data_dict["instrument_names"])
model.view_model(layout="dot")
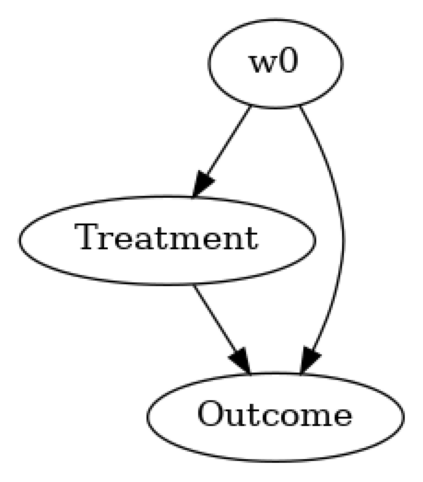
Showing the causal model stored in the local file “causal_model.png”
[5]:
from IPython.display import Image, display
display(Image(filename="causal_model.png"))
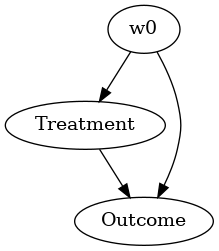
STEP 2: Identify causal effect using properties of the formal causal graph#
Identify the causal effect using properties of the causal graph.
[6]:
identified_estimand = model.identify_effect(proceed_when_unidentifiable=True)
print(identified_estimand)
Estimand type: EstimandType.NONPARAMETRIC_ATE
### Estimand : 1
Estimand name: backdoor
Estimand expression:
d
────────────(E[Outcome|w0])
d[Treatment]
Estimand assumption 1, Unconfoundedness: If U→{Treatment} and U→Outcome then P(Outcome|Treatment,w0,U) = P(Outcome|Treatment,w0)
### Estimand : 2
Estimand name: iv
No such variable(s) found!
### Estimand : 3
Estimand name: frontdoor
No such variable(s) found!
STEP 3: Estimate the causal effect#
Once we have identified the estimand, we can use any statistical method to estimate the causal effect.
Let’s use Linear Regression for simplicity.
[7]:
estimate = model.estimate_effect(identified_estimand,
method_name="backdoor.linear_regression")
print("Causal Estimate is " + str(estimate.value))
# Plot Slope of line between treamtent and outcome =causal effect
dowhy.plotter.plot_causal_effect(estimate, df[data_dict["treatment_name"]], df[data_dict["outcome_name"]])
Causal Estimate is 1.0121551154547133
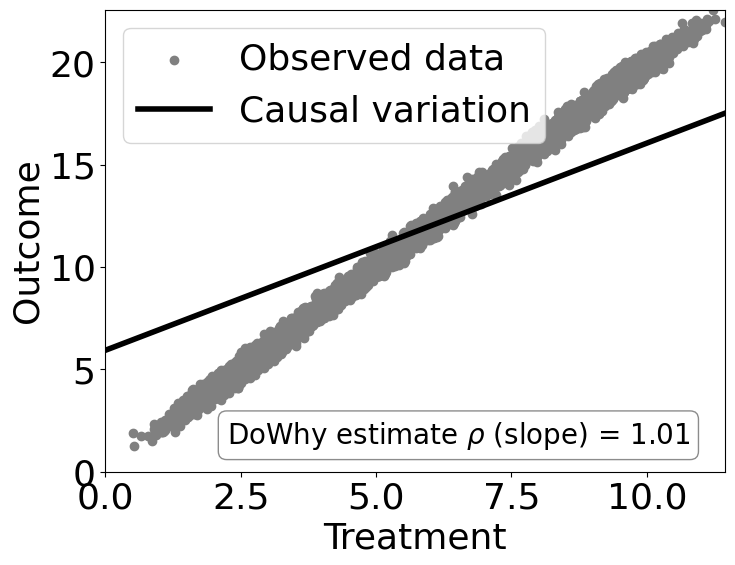
Checking if the estimate is correct#
[8]:
print("DoWhy estimate is " + str(estimate.value))
print ("Actual true causal effect was {0}".format(rvar))
DoWhy estimate is 1.0121551154547133
Actual true causal effect was 1
Step 4: Refuting the estimate#
We can also refute the estimate to check its robustness to assumptions (aka sensitivity analysis, but on steroids).
Adding a random common cause variable#
[9]:
res_random=model.refute_estimate(identified_estimand, estimate, method_name="random_common_cause")
print(res_random)
Refute: Add a random common cause
Estimated effect:1.0121551154547133
New effect:1.0121408959949347
p value:0.96
Replacing treatment with a random (placebo) variable#
[10]:
res_placebo=model.refute_estimate(identified_estimand, estimate,
method_name="placebo_treatment_refuter", placebo_type="permute")
print(res_placebo)
Refute: Use a Placebo Treatment
Estimated effect:1.0121551154547133
New effect:-7.908637019507836e-05
p value:1.0
Removing a random subset of the data#
[11]:
res_subset=model.refute_estimate(identified_estimand, estimate,
method_name="data_subset_refuter", subset_fraction=0.9)
print(res_subset)
Refute: Use a subset of data
Estimated effect:1.0121551154547133
New effect:1.0119332285840539
p value:0.96
As you can see, our causal estimator is robust to simple refutations.