Estimating effect of multiple treatments#
[1]:
from dowhy import CausalModel
import dowhy.datasets
import warnings
warnings.filterwarnings('ignore')
[2]:
data = dowhy.datasets.linear_dataset(10, num_common_causes=4, num_samples=10000,
num_instruments=0, num_effect_modifiers=2,
num_treatments=2,
treatment_is_binary=False,
num_discrete_common_causes=2,
num_discrete_effect_modifiers=0,
one_hot_encode=False)
df=data['df']
df.head()
[2]:
X0 | X1 | W0 | W1 | W2 | W3 | v0 | v1 | y | |
---|---|---|---|---|---|---|---|---|---|
0 | -0.610485 | -0.361963 | 0.454827 | 1.298354 | 0 | 1 | 8.448088 | 6.745871 | 41.409778 |
1 | -0.382653 | -0.207038 | 0.248333 | -1.312163 | 2 | 0 | 1.797379 | 3.629792 | 40.533986 |
2 | -2.544719 | 1.385429 | 0.878959 | -0.897343 | 2 | 1 | 5.830589 | 8.248788 | 97.279320 |
3 | -1.087287 | -0.360749 | -1.518408 | -2.218330 | 2 | 0 | -10.533392 | -4.248372 | -291.363295 |
4 | -0.611511 | -1.514700 | 1.302823 | -0.726144 | 3 | 2 | 12.254958 | 15.266457 | -704.334604 |
[3]:
model = CausalModel(data=data["df"],
treatment=data["treatment_name"], outcome=data["outcome_name"],
graph=data["gml_graph"])
[4]:
model.view_model()
from IPython.display import Image, display
display(Image(filename="causal_model.png"))
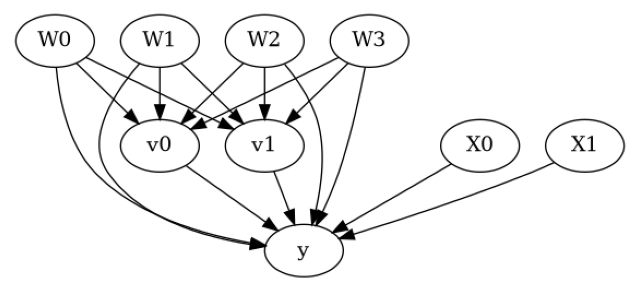
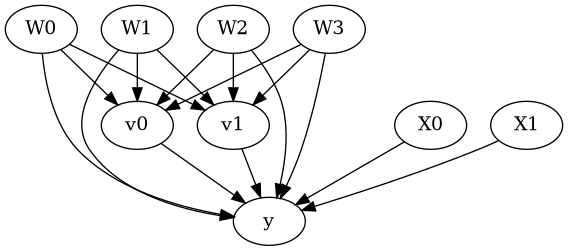
[5]:
identified_estimand= model.identify_effect(proceed_when_unidentifiable=True)
print(identified_estimand)
Estimand type: EstimandType.NONPARAMETRIC_ATE
### Estimand : 1
Estimand name: backdoor
Estimand expression:
d
─────────(E[y|W2,W3,W0,W1])
d[v₀ v₁]
Estimand assumption 1, Unconfoundedness: If U→{v0,v1} and U→y then P(y|v0,v1,W2,W3,W0,W1,U) = P(y|v0,v1,W2,W3,W0,W1)
### Estimand : 2
Estimand name: iv
No such variable(s) found!
### Estimand : 3
Estimand name: frontdoor
No such variable(s) found!
Linear model#
Let us first see an example for a linear model. The control_value and treatment_value can be provided as a tuple/list when the treatment is multi-dimensional.
The interpretation is change in y when v0 and v1 are changed from (0,0) to (1,1).
[6]:
linear_estimate = model.estimate_effect(identified_estimand,
method_name="backdoor.linear_regression",
control_value=(0,0),
treatment_value=(1,1),
method_params={'need_conditional_estimates': False})
print(linear_estimate)
*** Causal Estimate ***
## Identified estimand
Estimand type: EstimandType.NONPARAMETRIC_ATE
### Estimand : 1
Estimand name: backdoor
Estimand expression:
d
─────────(E[y|W2,W3,W0,W1])
d[v₀ v₁]
Estimand assumption 1, Unconfoundedness: If U→{v0,v1} and U→y then P(y|v0,v1,W2,W3,W0,W1,U) = P(y|v0,v1,W2,W3,W0,W1)
## Realized estimand
b: y~v0+v1+W2+W3+W0+W1+v0*X1+v0*X0+v1*X1+v1*X0
Target units: ate
## Estimate
Mean value: 26.688772806024613
You can estimate conditional effects, based on effect modifiers.
[7]:
linear_estimate = model.estimate_effect(identified_estimand,
method_name="backdoor.linear_regression",
control_value=(0,0),
treatment_value=(1,1))
print(linear_estimate)
*** Causal Estimate ***
## Identified estimand
Estimand type: EstimandType.NONPARAMETRIC_ATE
### Estimand : 1
Estimand name: backdoor
Estimand expression:
d
─────────(E[y|W2,W3,W0,W1])
d[v₀ v₁]
Estimand assumption 1, Unconfoundedness: If U→{v0,v1} and U→y then P(y|v0,v1,W2,W3,W0,W1,U) = P(y|v0,v1,W2,W3,W0,W1)
## Realized estimand
b: y~v0+v1+W2+W3+W0+W1+v0*X1+v0*X0+v1*X1+v1*X0
Target units:
## Estimate
Mean value: 26.688772806024613
### Conditional Estimates
__categorical__X1 __categorical__X0
(-3.26, -0.246] (-4.732, -1.371] -40.208441
(-1.371, -0.795] -25.115264
(-0.795, -0.303] -13.251766
(-0.303, 0.281] -4.487960
(0.281, 3.645] 13.324645
(-0.246, 0.352] (-4.732, -1.371] -16.542026
(-1.371, -0.795] 1.315167
(-0.795, -0.303] 11.386216
(-0.303, 0.281] 21.408088
(0.281, 3.645] 38.025938
(0.352, 0.862] (-4.732, -1.371] 0.328755
(-1.371, -0.795] 16.467108
(-0.795, -0.303] 26.378072
(-0.303, 0.281] 36.580765
(0.281, 3.645] 53.877811
(0.862, 1.453] (-4.732, -1.371] 15.220637
(-1.371, -0.795] 31.770172
(-0.795, -0.303] 42.066948
(-0.303, 0.281] 52.743631
(0.281, 3.645] 69.495885
(1.453, 4.515] (-4.732, -1.371] 41.171839
(-1.371, -0.795] 57.430537
(-0.795, -0.303] 67.502442
(-0.303, 0.281] 76.439040
(0.281, 3.645] 93.678987
dtype: float64
More methods#
You can also use methods from EconML or CausalML libraries that support multiple treatments. You can look at examples from the conditional effect notebook: https://py-why.github.io/dowhy/example_notebooks/dowhy-conditional-treatment-effects.html
Propensity-based methods do not support multiple treatments currently.