Demo for the DoWhy causal API#
We show a simple example of adding a causal extension to any dataframe.
[1]:
import dowhy.datasets
import dowhy.api
from dowhy.graph import build_graph_from_str
import numpy as np
import pandas as pd
from statsmodels.api import OLS
[2]:
data = dowhy.datasets.linear_dataset(beta=5,
num_common_causes=1,
num_instruments = 0,
num_samples=1000,
treatment_is_binary=True)
df = data['df']
df['y'] = df['y'] + np.random.normal(size=len(df)) # Adding noise to data. Without noise, the variance in Y|X, Z is zero, and mcmc fails.
nx_graph = build_graph_from_str(data["dot_graph"])
treatment= data["treatment_name"][0]
outcome = data["outcome_name"][0]
common_cause = data["common_causes_names"][0]
df
[2]:
W0 | v0 | y | |
---|---|---|---|
0 | 2.357083 | True | 9.125432 |
1 | 1.083218 | True | 8.333562 |
2 | -1.405914 | True | 0.612934 |
3 | 0.544866 | True | 7.162384 |
4 | 0.678475 | True | 5.906530 |
... | ... | ... | ... |
995 | 1.141585 | False | 1.742434 |
996 | 1.448861 | True | 9.645512 |
997 | -1.180041 | False | -2.199560 |
998 | 0.331246 | True | 4.966359 |
999 | -1.905690 | False | -5.905464 |
1000 rows × 3 columns
[3]:
# data['df'] is just a regular pandas.DataFrame
df.causal.do(x=treatment,
variable_types={treatment: 'b', outcome: 'c', common_cause: 'c'},
outcome=outcome,
common_causes=[common_cause],
).groupby(treatment).mean().plot(y=outcome, kind='bar')
[3]:
<Axes: xlabel='v0'>
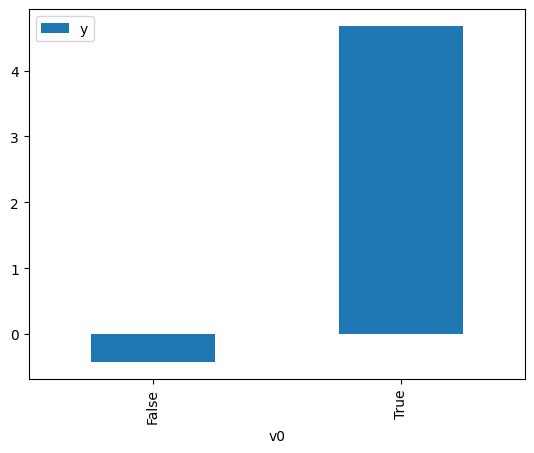
[4]:
df.causal.do(x={treatment: 1},
variable_types={treatment:'b', outcome: 'c', common_cause: 'c'},
outcome=outcome,
method='weighting',
common_causes=[common_cause]
).groupby(treatment).mean().plot(y=outcome, kind='bar')
[4]:
<Axes: xlabel='v0'>
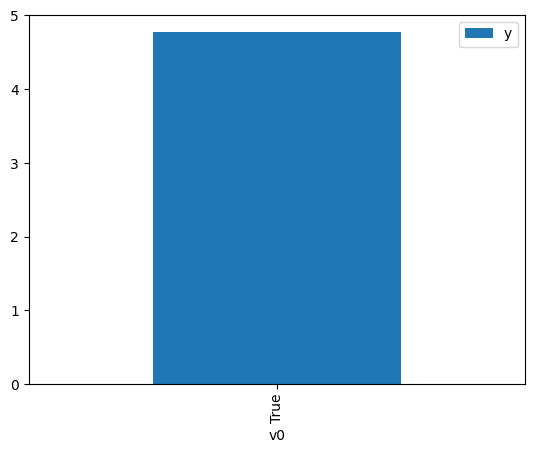
[5]:
cdf_1 = df.causal.do(x={treatment: 1},
variable_types={treatment: 'b', outcome: 'c', common_cause: 'c'},
outcome=outcome,
graph=nx_graph
)
cdf_0 = df.causal.do(x={treatment: 0},
variable_types={treatment: 'b', outcome: 'c', common_cause: 'c'},
outcome=outcome,
graph=nx_graph
)
[6]:
cdf_0
[6]:
W0 | v0 | y | propensity_score | weight | |
---|---|---|---|---|---|
0 | 1.863823 | False | 5.043438 | 0.341879 | 2.925007 |
1 | -0.942918 | False | -3.132091 | 0.628782 | 1.590376 |
2 | 2.051547 | False | 5.045559 | 0.324322 | 3.083357 |
3 | 0.272542 | False | 1.963705 | 0.503791 | 1.984952 |
4 | -0.626944 | False | -2.131502 | 0.597230 | 1.674395 |
... | ... | ... | ... | ... | ... |
995 | 0.274917 | False | 1.188372 | 0.503541 | 1.985937 |
996 | 2.142835 | False | 3.724875 | 0.315955 | 3.165004 |
997 | 1.085725 | False | 1.130440 | 0.418905 | 2.387177 |
998 | 1.057579 | False | 3.510709 | 0.421793 | 2.370833 |
999 | 0.407694 | False | 1.097901 | 0.489564 | 2.042634 |
1000 rows × 5 columns
[7]:
cdf_1
[7]:
W0 | v0 | y | propensity_score | weight | |
---|---|---|---|---|---|
0 | 0.700487 | True | 6.066825 | 0.541168 | 1.847855 |
1 | 3.784091 | True | 12.722615 | 0.812076 | 1.231411 |
2 | -0.491960 | True | 6.804122 | 0.416515 | 2.400874 |
3 | 2.036653 | True | 10.598381 | 0.674302 | 1.483014 |
4 | 0.350898 | True | 6.921556 | 0.504458 | 1.982325 |
... | ... | ... | ... | ... | ... |
995 | -0.334890 | True | 5.265809 | 0.432673 | 2.311215 |
996 | 0.624873 | True | 8.145840 | 0.533252 | 1.875286 |
997 | -0.635418 | True | 2.679304 | 0.401911 | 2.488111 |
998 | 1.072236 | True | 8.313301 | 0.579712 | 1.724995 |
999 | 0.011895 | True | 4.776788 | 0.468810 | 2.133060 |
1000 rows × 5 columns
Comparing the estimate to Linear Regression#
First, estimating the effect using the causal data frame, and the 95% confidence interval.
[8]:
(cdf_1['y'] - cdf_0['y']).mean()
[8]:
$\displaystyle 5.01140720806203$
[9]:
1.96*(cdf_1['y'] - cdf_0['y']).std() / np.sqrt(len(df))
[9]:
$\displaystyle 0.233198698453047$
Comparing to the estimate from OLS.
[10]:
model = OLS(np.asarray(df[outcome]), np.asarray(df[[common_cause, treatment]], dtype=np.float64))
result = model.fit()
result.summary()
[10]:
Dep. Variable: | y | R-squared (uncentered): | 0.966 |
---|---|---|---|
Model: | OLS | Adj. R-squared (uncentered): | 0.966 |
Method: | Least Squares | F-statistic: | 1.425e+04 |
Date: | Fri, 22 Nov 2024 | Prob (F-statistic): | 0.00 |
Time: | 16:01:30 | Log-Likelihood: | -1453.3 |
No. Observations: | 1000 | AIC: | 2911. |
Df Residuals: | 998 | BIC: | 2920. |
Df Model: | 2 | ||
Covariance Type: | nonrobust |
coef | std err | t | P>|t| | [0.025 | 0.975] | |
---|---|---|---|---|---|---|
x1 | 2.4043 | 0.033 | 73.345 | 0.000 | 2.340 | 2.469 |
x2 | 5.0004 | 0.052 | 96.720 | 0.000 | 4.899 | 5.102 |
Omnibus: | 0.868 | Durbin-Watson: | 1.919 |
---|---|---|---|
Prob(Omnibus): | 0.648 | Jarque-Bera (JB): | 0.742 |
Skew: | 0.003 | Prob(JB): | 0.690 |
Kurtosis: | 3.133 | Cond. No. | 2.05 |
Notes:
[1] R² is computed without centering (uncentered) since the model does not contain a constant.
[2] Standard Errors assume that the covariance matrix of the errors is correctly specified.