DoWhy: Interpreters for Causal Estimators#
This is a quick introduction to the use of interpreters in the DoWhy causal inference library. We will load in a sample dataset, use different methods for estimating the causal effect of a (pre-specified)treatment variable on a (pre-specified) outcome variable and demonstrate how to interpret the obtained results.
First, let us add the required path for Python to find the DoWhy code and load all required packages
[1]:
%load_ext autoreload
%autoreload 2
[2]:
import numpy as np
import pandas as pd
import logging
import dowhy
from dowhy import CausalModel
import dowhy.datasets
Now, let us load a dataset. For simplicity, we simulate a dataset with linear relationships between common causes and treatment, and common causes and outcome.
Beta is the true causal effect.
[3]:
data = dowhy.datasets.linear_dataset(beta=1,
num_common_causes=5,
num_instruments = 2,
num_treatments=1,
num_discrete_common_causes=1,
num_samples=10000,
treatment_is_binary=True,
outcome_is_binary=False)
df = data["df"]
print(df[df.v0==True].shape[0])
df
8729
[3]:
Z0 | Z1 | W0 | W1 | W2 | W3 | W4 | v0 | y | |
---|---|---|---|---|---|---|---|---|---|
0 | 1.0 | 0.219384 | 1.990344 | 0.304821 | -0.050378 | 1.068891 | 2 | True | 2.624553 |
1 | 0.0 | 0.056212 | 0.658407 | -0.521509 | 1.846107 | -0.887286 | 1 | True | 3.021249 |
2 | 0.0 | 0.855904 | -0.531855 | -0.632133 | 1.167153 | -1.104222 | 2 | True | 2.385235 |
3 | 0.0 | 0.427827 | 2.517520 | 0.581278 | 1.377727 | -0.420942 | 1 | True | 3.704422 |
4 | 0.0 | 0.016795 | 1.617643 | 0.636910 | 0.388238 | 2.358816 | 3 | True | 3.659491 |
... | ... | ... | ... | ... | ... | ... | ... | ... | ... |
9995 | 0.0 | 0.555651 | 0.715430 | 0.061413 | 0.627921 | -0.461876 | 3 | True | 3.089769 |
9996 | 0.0 | 0.128355 | 0.075753 | 0.858358 | 0.949139 | -0.648450 | 1 | True | 2.699778 |
9997 | 0.0 | 0.785210 | 0.537969 | -0.299576 | 0.106961 | 1.365880 | 2 | True | 2.098799 |
9998 | 0.0 | 0.298603 | 1.858316 | 1.303487 | -0.464985 | 0.764863 | 1 | True | 2.252817 |
9999 | 0.0 | 0.316337 | -0.819686 | 0.777202 | 1.568057 | 1.500237 | 0 | False | 1.756179 |
10000 rows × 9 columns
Note that we are using a pandas dataframe to load the data.
Identifying the causal estimand#
We now input a causal graph in the GML graph format.
[4]:
# With graph
model=CausalModel(
data = df,
treatment=data["treatment_name"],
outcome=data["outcome_name"],
graph=data["gml_graph"],
instruments=data["instrument_names"]
)
[5]:
model.view_model()
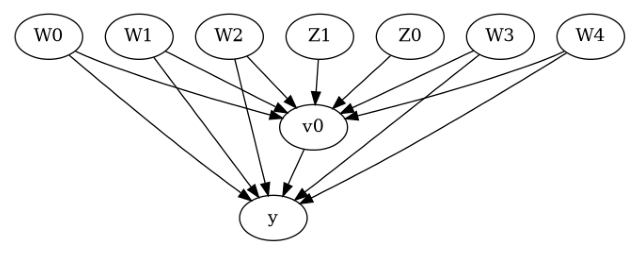
[6]:
from IPython.display import Image, display
display(Image(filename="causal_model.png"))
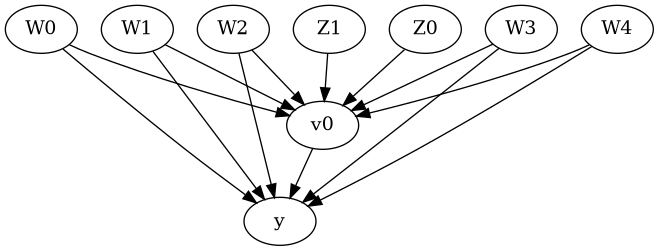
We get a causal graph. Now identification and estimation is done.
[7]:
identified_estimand = model.identify_effect(proceed_when_unidentifiable=True)
print(identified_estimand)
Estimand type: EstimandType.NONPARAMETRIC_ATE
### Estimand : 1
Estimand name: backdoor
Estimand expression:
d
─────(E[y|W1,W3,W2,W4,W0])
d[v₀]
Estimand assumption 1, Unconfoundedness: If U→{v0} and U→y then P(y|v0,W1,W3,W2,W4,W0,U) = P(y|v0,W1,W3,W2,W4,W0)
### Estimand : 2
Estimand name: iv
Estimand expression:
⎡ -1⎤
⎢ d ⎛ d ⎞ ⎥
E⎢─────────(y)⋅⎜─────────([v₀])⎟ ⎥
⎣d[Z₀ Z₁] ⎝d[Z₀ Z₁] ⎠ ⎦
Estimand assumption 1, As-if-random: If U→→y then ¬(U →→{Z0,Z1})
Estimand assumption 2, Exclusion: If we remove {Z0,Z1}→{v0}, then ¬({Z0,Z1}→y)
### Estimand : 3
Estimand name: frontdoor
No such variable(s) found!
Method 1: Propensity Score Stratification#
We will be using propensity scores to stratify units in the data.
[8]:
causal_estimate_strat = model.estimate_effect(identified_estimand,
method_name="backdoor.propensity_score_stratification",
target_units="att")
print(causal_estimate_strat)
print("Causal Estimate is " + str(causal_estimate_strat.value))
*** Causal Estimate ***
## Identified estimand
Estimand type: EstimandType.NONPARAMETRIC_ATE
### Estimand : 1
Estimand name: backdoor
Estimand expression:
d
─────(E[y|W1,W3,W2,W4,W0])
d[v₀]
Estimand assumption 1, Unconfoundedness: If U→{v0} and U→y then P(y|v0,W1,W3,W2,W4,W0,U) = P(y|v0,W1,W3,W2,W4,W0)
## Realized estimand
b: y~v0+W1+W3+W2+W4+W0
Target units: att
## Estimate
Mean value: 0.9964020206522214
Causal Estimate is 0.9964020206522214
Textual Interpreter#
The textual Interpreter describes (in words) the effect of unit change in the treatment variable on the outcome variable.
[9]:
# Textual Interpreter
interpretation = causal_estimate_strat.interpret(method_name="textual_effect_interpreter")
Increasing the treatment variable(s) [v0] from 0 to 1 causes an increase of 0.9964020206522214 in the expected value of the outcome [['y']], over the data distribution/population represented by the dataset.
Visual Interpreter#
The visual interpreter plots the change in the standardized mean difference (SMD) before and after Propensity Score based adjustment of the dataset. The formula for SMD is given below.
\(SMD = \frac{\bar X_{1} - \bar X_{2}}{\sqrt{(S_{1}^{2} + S_{2}^{2})/2}}\)
Here, \(\bar X_{1}\) and \(\bar X_{2}\) are the sample mean for the treated and control groups.
[10]:
# Visual Interpreter
interpretation = causal_estimate_strat.interpret(method_name="propensity_balance_interpreter")
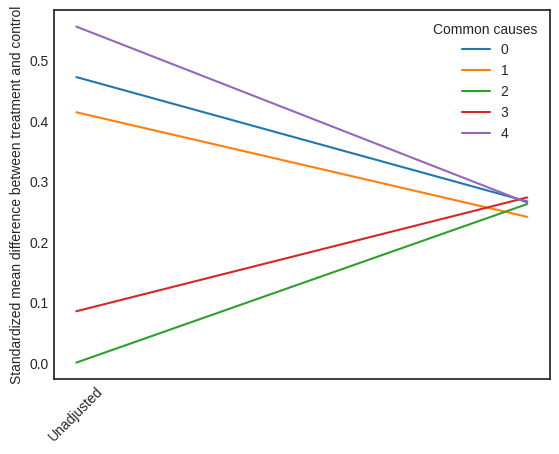
This plot shows how the SMD decreases from the unadjusted to the stratified units.
Method 2: Propensity Score Matching#
We will be using propensity scores to match units in the data.
[11]:
causal_estimate_match = model.estimate_effect(identified_estimand,
method_name="backdoor.propensity_score_matching",
target_units="atc")
print(causal_estimate_match)
print("Causal Estimate is " + str(causal_estimate_match.value))
*** Causal Estimate ***
## Identified estimand
Estimand type: EstimandType.NONPARAMETRIC_ATE
### Estimand : 1
Estimand name: backdoor
Estimand expression:
d
─────(E[y|W1,W3,W2,W4,W0])
d[v₀]
Estimand assumption 1, Unconfoundedness: If U→{v0} and U→y then P(y|v0,W1,W3,W2,W4,W0,U) = P(y|v0,W1,W3,W2,W4,W0)
## Realized estimand
b: y~v0+W1+W3+W2+W4+W0
Target units: atc
## Estimate
Mean value: 1.027917697092231
Causal Estimate is 1.027917697092231
[12]:
# Textual Interpreter
interpretation = causal_estimate_match.interpret(method_name="textual_effect_interpreter")
Increasing the treatment variable(s) [v0] from 0 to 1 causes an increase of 1.027917697092231 in the expected value of the outcome [['y']], over the data distribution/population represented by the dataset.
Cannot use propensity balance interpretor here since the interpreter method only supports propensity score stratification estimator.
Method 3: Weighting#
We will be using (inverse) propensity scores to assign weights to units in the data. DoWhy supports a few different weighting schemes:
Vanilla Inverse Propensity Score weighting (IPS) (weighting_scheme=”ips_weight”)
Self-normalized IPS weighting (also known as the Hajek estimator) (weighting_scheme=”ips_normalized_weight”)
Stabilized IPS weighting (weighting_scheme = “ips_stabilized_weight”)
[13]:
causal_estimate_ipw = model.estimate_effect(identified_estimand,
method_name="backdoor.propensity_score_weighting",
target_units = "ate",
method_params={"weighting_scheme":"ips_weight"})
print(causal_estimate_ipw)
print("Causal Estimate is " + str(causal_estimate_ipw.value))
*** Causal Estimate ***
## Identified estimand
Estimand type: EstimandType.NONPARAMETRIC_ATE
### Estimand : 1
Estimand name: backdoor
Estimand expression:
d
─────(E[y|W1,W3,W2,W4,W0])
d[v₀]
Estimand assumption 1, Unconfoundedness: If U→{v0} and U→y then P(y|v0,W1,W3,W2,W4,W0,U) = P(y|v0,W1,W3,W2,W4,W0)
## Realized estimand
b: y~v0+W1+W3+W2+W4+W0
Target units: ate
## Estimate
Mean value: 1.42026175044465
Causal Estimate is 1.42026175044465
[14]:
# Textual Interpreter
interpretation = causal_estimate_ipw.interpret(method_name="textual_effect_interpreter")
Increasing the treatment variable(s) [v0] from 0 to 1 causes an increase of 1.42026175044465 in the expected value of the outcome [['y']], over the data distribution/population represented by the dataset.
[15]:
interpretation = causal_estimate_ipw.interpret(method_name="confounder_distribution_interpreter", fig_size=(8,8), font_size=12, var_name='W4', var_type='discrete')
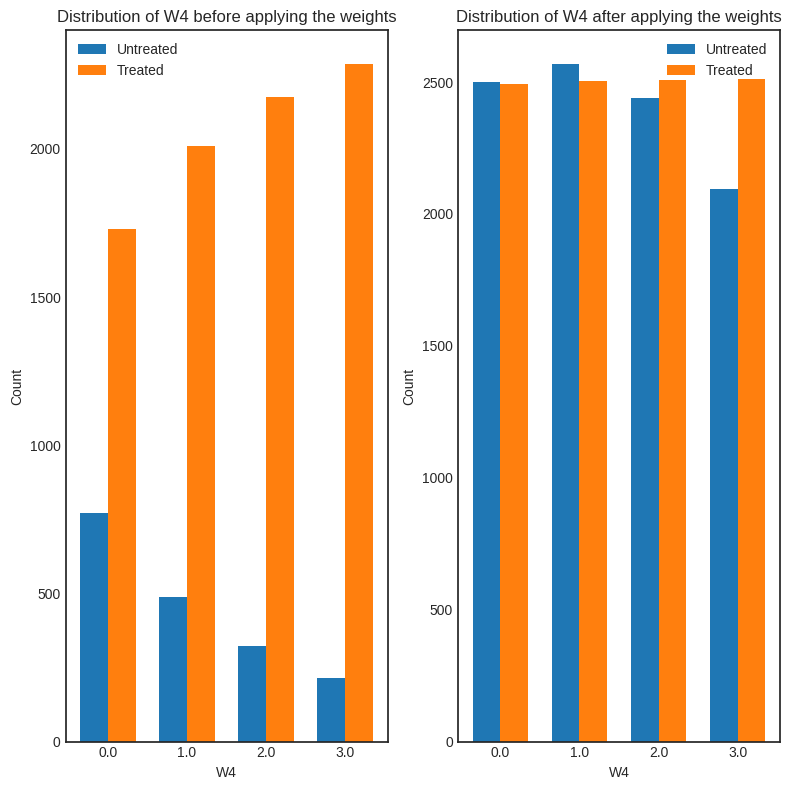
[ ]: