3.1.11. pywhy_graphs.networkx.m_separated#
- pywhy_graphs.networkx.m_separated(G, x, y, z, directed_edge_name='directed', bidirected_edge_name='bidirected', undirected_edge_name='undirected')[source]#
Check m-separation among ‘x’ and ‘y’ given ‘z’ in mixed-edge causal graph G, which may contain directed, bidirected, and undirected edges.
This implements the m-separation algorithm TESTSEP presented in [1] for ancestral mixed graphs. Further checks have ensure that it works for non-ancestral mixed graphs (e.g. ADMGs). The algorithm performs a breadth-first search over m-connecting paths between ‘x’ and ‘y’ (i.e. a path on which every node that is a collider is in ‘z’, and every node that is not a collider is not in ‘z’). The algorithm has runtime \(O(|E| + |V|)\) for number of edges \(|E|\) and number of vertices \(|V|\).
- Parameters:
- Gmixed-edge-graph
Mixed edge causal graph.
- xset
First set of nodes in
G
.- yset
Second set of nodes in
G
.- zset
Set of conditioning nodes in
G
. Can be empty set.- directed_edge_namestr
Name of the directed edge, default is directed.
- bidirected_edge_namestr
Name of the bidirected edge, default is bidirected.
- undirected_edge_namestr
Name of the undirected edge, default is undirected.
- Returns:
- bbool
A boolean that is true if
x
is m-separated fromy
givenz
inG
.
Notes
This wraps the networkx implementation, which only allows DAGs and does not have an
ADMG
representation.References
[1]B. van der Zander, M. Liśkiewicz, and J. Textor, “Separators and Adjustment Sets in Causal Graphs: Complete Criteria and an Algorithmic Framework,” Artificial Intelligence, vol. 270, pp. 1–40, May 2019, doi: 10.1016/j.artint.2018.12.006.
3.1.11.1. Examples using pywhy_graphs.networkx.m_separated
#
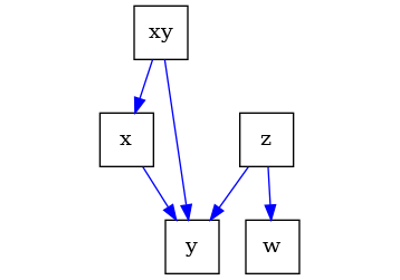
An introduction to causal graphs and how to use them