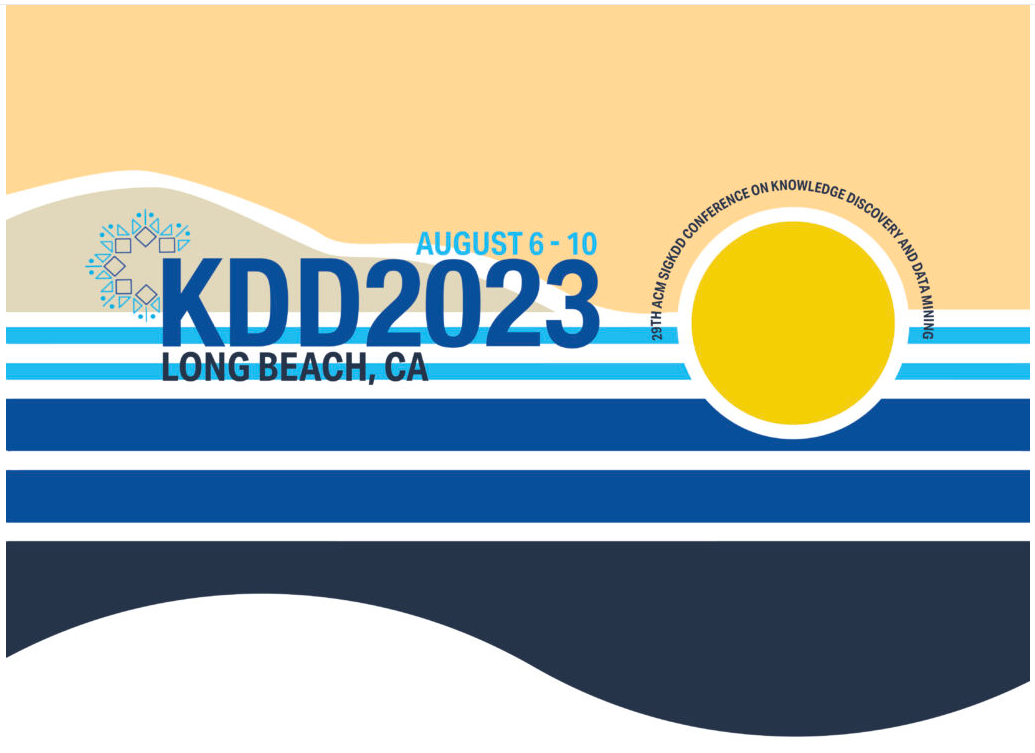
This workshop aims to bring together researchers and practitioners from academia and industry to share their experiences and insights on applying causal inference and machine learning techniques to real-world problems in the areas of product, brand, policy, and beyond. The workshop welcomes original research that covers machine learning theory, deep learning, causal inference, and online learning. Additionally, the workshop encourages topics that address scalable system design, algorithm bias, and interpretability.
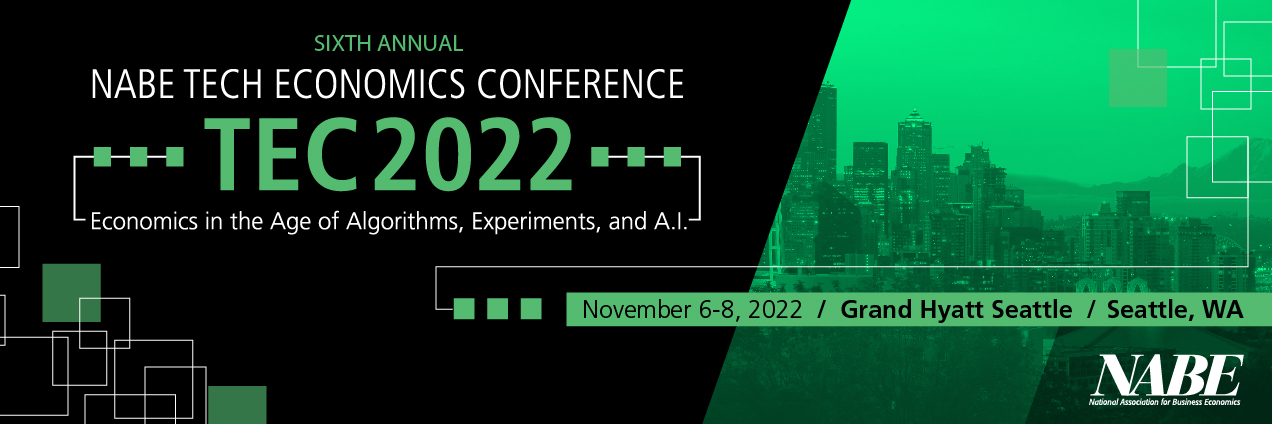
For decades, causal inference methods have found wide applicability in the social and biomedical sciences. As computing systems start intervening in our work and daily lives, questions of cause-and-effect are gaining importance in computer science as well. To enable widespread use of causal inference, we are pleased to announce a new software library, DoWhy.
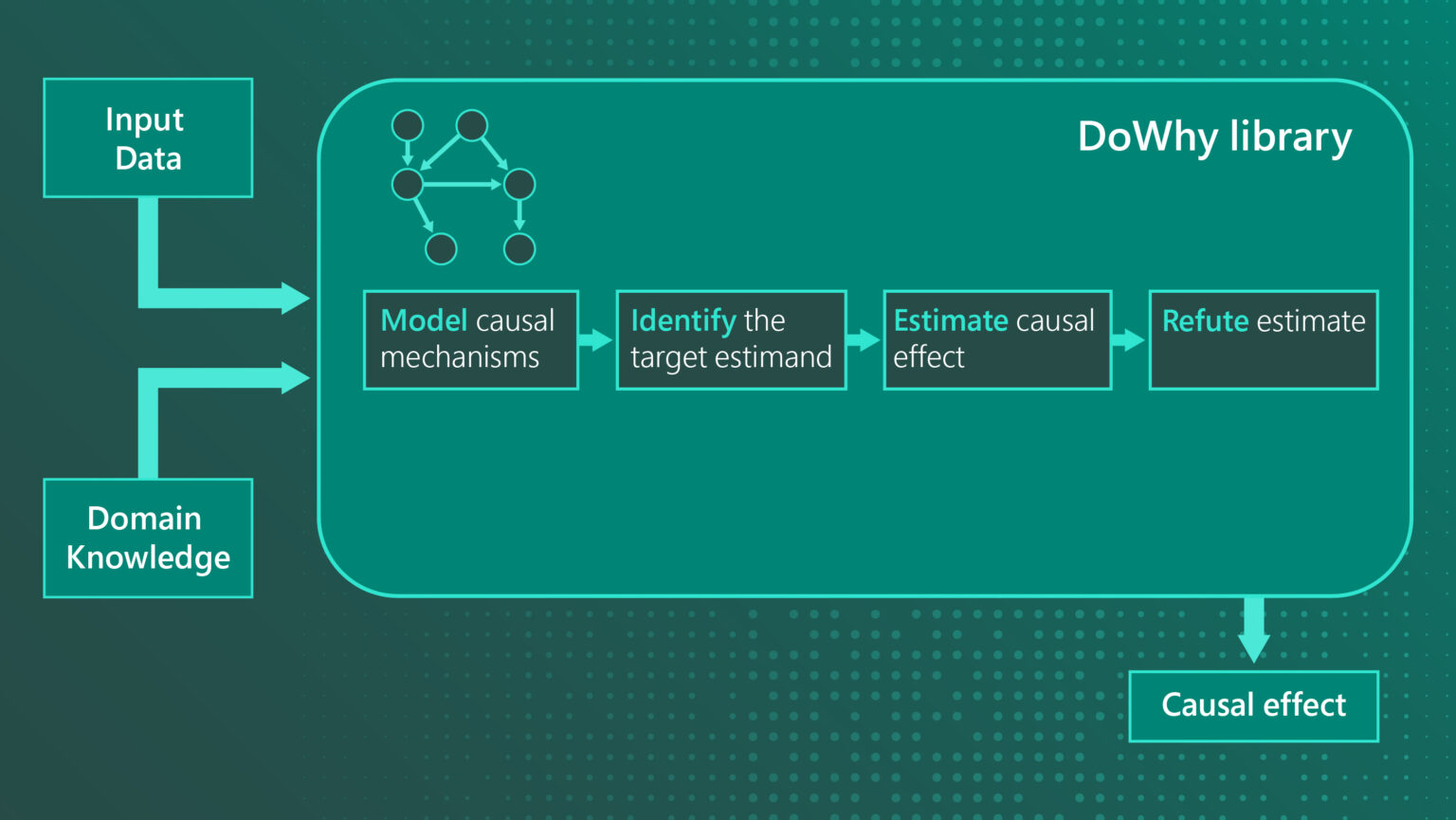
Identifying causal effects is an integral part of scientific inquiry. It helps us understand everything from educational outcomes to the effects of social policies to risk factors for diseases. Questions of cause-and-effect are also critical for the design and data-driven evaluation of many technological systems we build today.
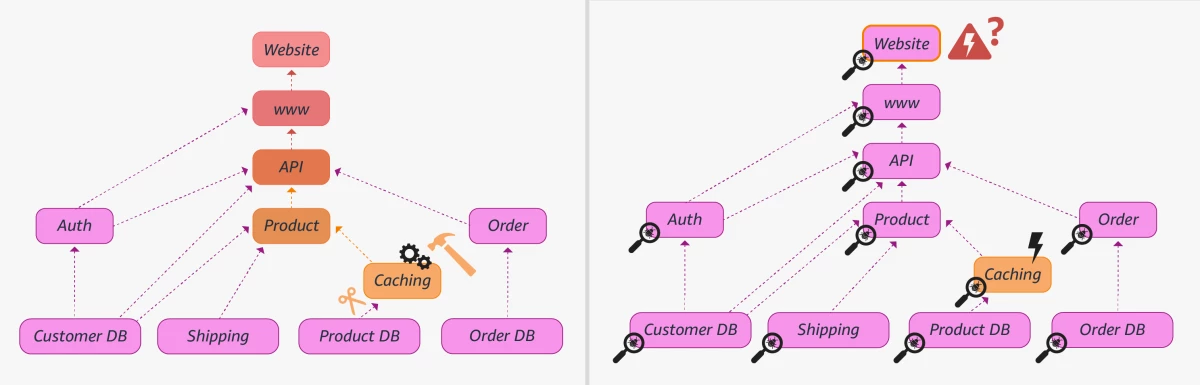
New features go beyond conventional effect estimation by attributing events to individual components of complex systems.
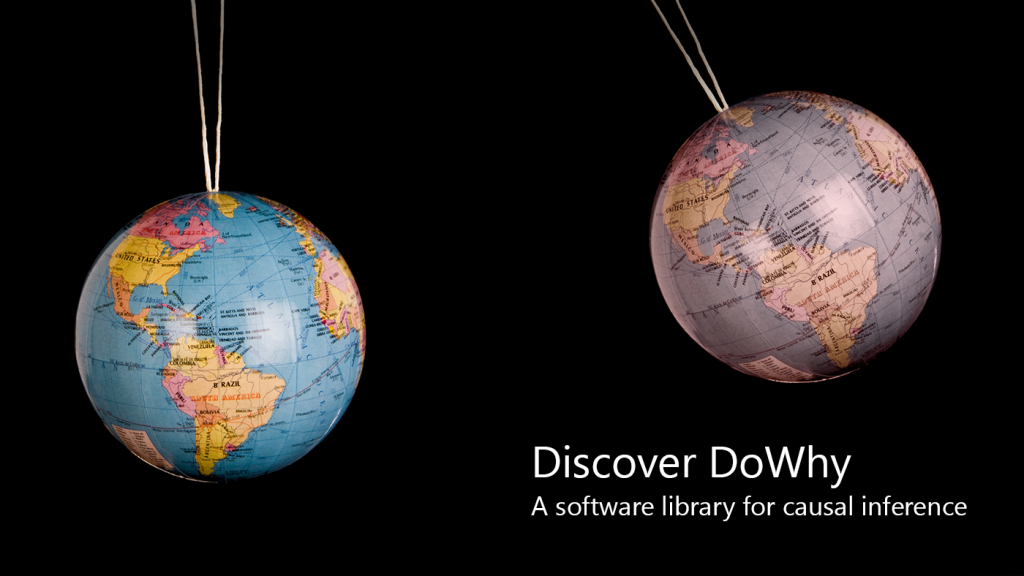
For decades, causal inference methods have found wide applicability in the social and biomedical sciences. As computing systems start intervening in our work and daily lives, questions of cause-and-effect are gaining importance in computer science as well. To enable widespread use of causal inference, we are pleased to announce a new software library, DoWhy.