Demo for the DoWhy causal API
We show a simple example of adding a causal extension to any dataframe.
[1]:
import dowhy.datasets
import dowhy.api
import numpy as np
import pandas as pd
from statsmodels.api import OLS
[2]:
data = dowhy.datasets.linear_dataset(beta=5,
num_common_causes=1,
num_instruments = 0,
num_samples=1000,
treatment_is_binary=True)
df = data['df']
df['y'] = df['y'] + np.random.normal(size=len(df)) # Adding noise to data. Without noise, the variance in Y|X, Z is zero, and mcmc fails.
#data['dot_graph'] = 'digraph { v ->y;X0-> v;X0-> y;}'
treatment= data["treatment_name"][0]
outcome = data["outcome_name"][0]
common_cause = data["common_causes_names"][0]
df
[2]:
W0 | v0 | y | |
---|---|---|---|
0 | -1.012777 | False | -3.042986 |
1 | 0.289858 | False | 1.112130 |
2 | 1.347723 | True | 8.063621 |
3 | -0.464752 | False | -0.189419 |
4 | 0.121820 | False | -0.439537 |
... | ... | ... | ... |
995 | -1.797895 | False | -4.532083 |
996 | -1.601010 | False | -4.809766 |
997 | -0.639639 | False | -1.936961 |
998 | -2.256174 | False | -5.319564 |
999 | -0.270505 | False | -0.054673 |
1000 rows × 3 columns
[3]:
# data['df'] is just a regular pandas.DataFrame
df.causal.do(x=treatment,
variable_types={treatment: 'b', outcome: 'c', common_cause: 'c'},
outcome=outcome,
common_causes=[common_cause],
proceed_when_unidentifiable=True).groupby(treatment).mean().plot(y=outcome, kind='bar')
[3]:
<Axes: xlabel='v0'>
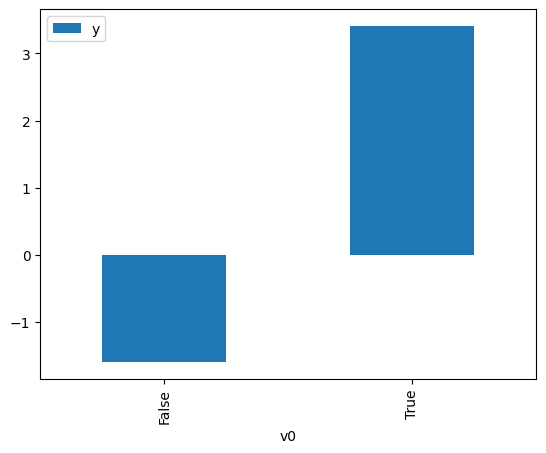
[4]:
df.causal.do(x={treatment: 1},
variable_types={treatment:'b', outcome: 'c', common_cause: 'c'},
outcome=outcome,
method='weighting',
common_causes=[common_cause],
proceed_when_unidentifiable=True).groupby(treatment).mean().plot(y=outcome, kind='bar')
[4]:
<Axes: xlabel='v0'>
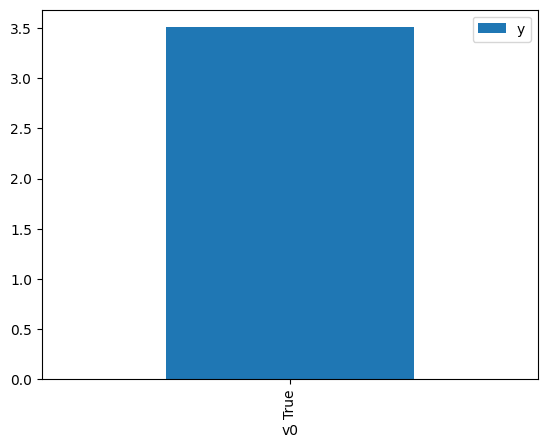
[5]:
cdf_1 = df.causal.do(x={treatment: 1},
variable_types={treatment: 'b', outcome: 'c', common_cause: 'c'},
outcome=outcome,
dot_graph=data['dot_graph'],
proceed_when_unidentifiable=True)
cdf_0 = df.causal.do(x={treatment: 0},
variable_types={treatment: 'b', outcome: 'c', common_cause: 'c'},
outcome=outcome,
dot_graph=data['dot_graph'],
proceed_when_unidentifiable=True)
[6]:
cdf_0
[6]:
W0 | v0 | y | propensity_score | weight | |
---|---|---|---|---|---|
0 | -0.304858 | False | -1.448515 | 0.532390 | 1.878323 |
1 | -0.625992 | False | -2.489382 | 0.594945 | 1.680829 |
2 | 0.918621 | False | 2.341392 | 0.301398 | 3.317870 |
3 | -0.650677 | False | -1.249433 | 0.599654 | 1.667629 |
4 | 0.232127 | False | 0.969079 | 0.426496 | 2.344690 |
... | ... | ... | ... | ... | ... |
995 | -0.486277 | False | -1.746970 | 0.567984 | 1.760612 |
996 | -0.994063 | False | -0.910734 | 0.662929 | 1.508456 |
997 | -0.600021 | False | -0.240069 | 0.589971 | 1.694998 |
998 | -1.467687 | False | -3.689877 | 0.741164 | 1.349230 |
999 | 0.139491 | False | -0.503729 | 0.444556 | 2.249433 |
1000 rows × 5 columns
[7]:
cdf_1
[7]:
W0 | v0 | y | propensity_score | weight | |
---|---|---|---|---|---|
0 | -0.540636 | True | 3.159776 | 0.421469 | 2.372652 |
1 | -0.517413 | True | 4.373290 | 0.425967 | 2.347601 |
2 | -1.011418 | True | 3.547779 | 0.334002 | 2.993996 |
3 | -1.705678 | True | -0.387705 | 0.224299 | 4.458329 |
4 | -1.809141 | True | -0.779102 | 0.210345 | 4.754091 |
... | ... | ... | ... | ... | ... |
995 | -0.207755 | True | 5.120488 | 0.486822 | 2.054139 |
996 | -0.597498 | True | 3.878264 | 0.410513 | 2.435976 |
997 | 0.806819 | True | 7.124728 | 0.679609 | 1.471435 |
998 | -1.339661 | True | 3.927840 | 0.278788 | 3.586958 |
999 | -2.158812 | True | -0.987639 | 0.167956 | 5.953941 |
1000 rows × 5 columns
Comparing the estimate to Linear Regression
First, estimating the effect using the causal data frame, and the 95% confidence interval.
[8]:
(cdf_1['y'] - cdf_0['y']).mean()
[8]:
$\displaystyle 5.00995911537052$
[9]:
1.96*(cdf_1['y'] - cdf_0['y']).std() / np.sqrt(len(df))
[9]:
$\displaystyle 0.214614822056212$
Comparing to the estimate from OLS.
[10]:
model = OLS(np.asarray(df[outcome]), np.asarray(df[[common_cause, treatment]], dtype=np.float64))
result = model.fit()
result.summary()
[10]:
Dep. Variable: | y | R-squared (uncentered): | 0.938 |
---|---|---|---|
Model: | OLS | Adj. R-squared (uncentered): | 0.938 |
Method: | Least Squares | F-statistic: | 7593. |
Date: | Tue, 05 Sep 2023 | Prob (F-statistic): | 0.00 |
Time: | 05:11:13 | Log-Likelihood: | -1418.2 |
No. Observations: | 1000 | AIC: | 2840. |
Df Residuals: | 998 | BIC: | 2850. |
Df Model: | 2 | ||
Covariance Type: | nonrobust |
coef | std err | t | P>|t| | [0.025 | 0.975] | |
---|---|---|---|---|---|---|
x1 | 2.3582 | 0.028 | 85.636 | 0.000 | 2.304 | 2.412 |
x2 | 5.0888 | 0.050 | 101.283 | 0.000 | 4.990 | 5.187 |
Omnibus: | 5.587 | Durbin-Watson: | 2.044 |
---|---|---|---|
Prob(Omnibus): | 0.061 | Jarque-Bera (JB): | 5.476 |
Skew: | -0.177 | Prob(JB): | 0.0647 |
Kurtosis: | 3.080 | Cond. No. | 1.87 |
Notes:
[1] R² is computed without centering (uncentered) since the model does not contain a constant.
[2] Standard Errors assume that the covariance matrix of the errors is correctly specified.